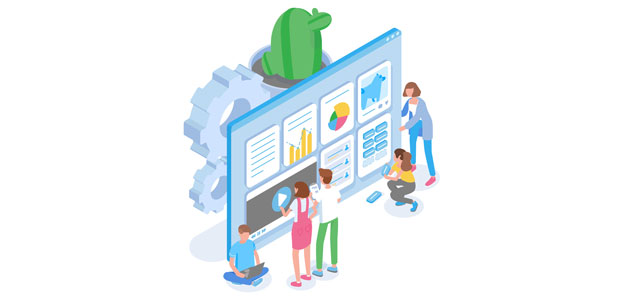
How to build a successful Enterprise-wide Data Strategy
Online streaming giant Netflix, which has over 150 million paid subscribers in over 190 countries, collects data from its users. Using advanced analytics to understand customer behaviour and buying patterns, the company makes extremely relevant customer recommendations, helping it achieve an impressive 93% customer retention.
Similarly, retail behemoth Walmart collects 2.5 petabytes of unstructured data from its 1 million customers every hour. This data helps the company arrive at a dynamic real time pricing model that has played a huge role in spurring its sales and increasing its revenues manifold in the last few years.
Both these cases highlight how using data effectively can make firms more competitive. The proficient use of data can help enterprises save money, improve business processes, increase customer-centricity, and develop ground-breaking marketing strategies. It can also enhance operational efficiency, mitigate risks, and generate new revenue models.
Therefore, it is hardly surprising that corporates are rushing to embrace data analytics solutions. A Frost and Sullivan report estimates that by 2023, the advanced analytics market will be worth $40.6bn, with a compound annual growth rate of 29.7%.
Challenges to adoption
Before enterprises jump headlong into implementing data analytics, they need to first undertake reasonable groundwork to ready the organisation. Efforts at implementing data analytics successfully are often hampered by the lack of pervasion of a data-driven culture, paucity of deep analytical and technical skills, and limited implementation experience.
The Infosys 'Digital Radar 2019: Barriers and Accelerators for Digital Transformation' report found that firms often implement digital technologies without appreciating inherent organisational challenges. As a result, they come away frustrated from the experience since the results do not match up to the promise.
Therefore, it is important to build a robust data- strategy before implementing any analytics initiatives. There are four aspects to this:
1) Culture
To build a data-driven culture, organisations must first hire skilled data scientists who have the requisite business domain knowledge along with the technical prowess. Next, they have to rethink their organisational pyramid such that data scientists are empowered to proliferate the data culture across the organisation.
Data scientists could help popularise the data culture and build awareness through initiatives such as employee-run hackathons or data-thons. Democratising data science by implementing self-service platforms could play a game-changing role within the organisation.
2) Leadership buy-in
In order to make any enduring organisational change it needs to be sponsored by senior leadership. It takes more than just executive buy-in. It is critical that key leaders across enterprise functions fully embrace data-led decisions and set the culture through examples. Only then will it cascade down to teams.
One great example is that of a British oil and gas company that developed a data marketplace to enable internal sharing and consumption of data. This not only improved data discovery but also had a positive impact on security and compliance.
Given that data analytics projects are often experimental in nature, leaders need to closely monitor results to determine if the efforts are aligned to strategic objectives.
3) Garnering quick wins
One of the best ways to create confidence in data-driven culture is to build strategies that deliver quick results, whether it is improved operational performance, higher levels of customer-centricity or new-found profits in non-traditional markets. Using design thinking techniques can help identify low hanging fruits and look for data-led themes that can be explored initially.
User experience will be an important driver here. These high visibility projects, when successful, can help convert sceptics within the organisation and garner greater support for larger initiatives.
4) Free up human intelligence
As data analytics becomes mature, it forms the foundation for more automation and artificial intelligence applications, further threatening the traditional roles of employees. However, rather than replacing human intelligence, these applications are much more likely to free employees from mundane, repetitive tasks.
For instance, chatbots or action bots can help automate several routine tasks such as data logging or answering basic customer queries. As a result, human intelligence is being freed up to focus on softer and more creative skills. Analytics and artificial intelligence solutions will inevitably support human intelligence to deliver the best results for the organisation. We are likely to see a move toward AI-assisted solutions rather than complete automation.
It is important to recognise that the end result of a strong data strategy is to build a knowledge-driven organisation where agility is an integral part of its DNA. Such an organisation works on an operating model that revolves around the employee and the customer, which equips it with the ability to quickly sense changing business needs and continuously evolve in response. Our point of view titled ‘The four pillars of an enterprise-wide data strategy’ shares several noteworthy insights on this.
A strong business core must be built up where all stakeholders can talk to each other in real-time, with intelligence and at the speed of data transmission, while also ensuring privacy and security. Once this is achieved, enterprises can hope to monetise their data effectively and deliver the best results for their customers.