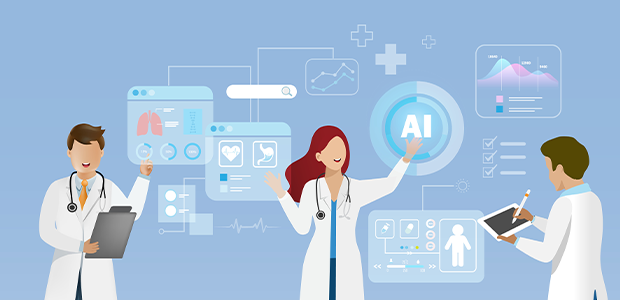
AI’s role in medical imaging for earlier anomaly detection
The hype surrounding AI remains prevalent in healthcare but is particularly strong in radiology. If you remember the early days of computer-aided design (CAD), it’s quite impressive how far the technology has come. A native of ChatGPT would perhaps contend that much work needs to be done before AI can reach its full potential in this field. Both views are correct. This article will examine why it is so difficult for AI to detect things, how its role is changing, and what trends to watch in 2025 and beyond.
Finding a needle in a haystack: detection is tough
Detecting disease early is difficult because diseases often start with rather subtle deviations from normal appearance in radiological imaging data. Because there is a lot of completely normal, natural variability between individuals, it’s very hard to determine which minor changes are truly abnormal. For instance, lung nodules start off very small; diffuse lung diseases begin with easily-overlooked tissue changes.
That is where Machine Learning (ML) plays an important role. It can learn to recognise the specific changes that are not normal, but rather associated with disease and separate them from normal variability. This normal variability can have different sources: individual anatomy, technical differences in the image acquisition equipment, or even temporal changes in tissue appearance that are perfectly normal. We need to train ML models with large amounts of data so that they can form representations of this variability and identify those changes that point to disease.
Can AI help us detect anomalies sooner?
AI can help in several ways. First, it can recognise specific patterns that are associated with disease, such as cancer, interstitial lung diseases, or cardiovascular disease in imaging data. By training on as diverse data as possible, AI is able to robustly detect findings that are important for the first diagnosis. And by parsing entire image volumes, it can support radiologists by highlighting suspicious areas, thereby increasing physicians’ sensitivity.
Secondly, AI can use image features beyond those that humans can easily observe and report. In lung cancer detection, radiologists first assess the size, shape, and category of a nodule to decide upon the next action in patient management. AI can analyse three-dimensional texture and fine-grained characteristics of a nodule’s surface to more reliably determine whether it carries a high or low risk of malignancy. This has direct consequences in the management of individual patients, such as whether or not that person will be sent for biopsy, or the length and frequency of follow-up intervals.
In a study by Adams et al. (JACR), it was shown that pairing guideline-based management of incidental nodules in chest CTs with ML-based analysis could significantly reduce false positives. This translates into both a reduced number of unnecessary biopsies (for the cases where the AI says the nodule is benign) and faster time to treatment (for the cases where the AI says the nodule is malignant). Here it is important to stress – AI is not advocating for the elimination of guidelines. Instead, we are being challenged to complement the necessary guidelines with AI results. In this case, if the ML score contradicts the guideline with high certainty, then go with the ML score; otherwise stick with the guideline instructions. We will see more applications like this in the future.
Thirdly, AI can help to quantify change over time in patients, which is again, crucial for proper follow-up. Current algorithms in the area of ML and medical image analysis can align multiple images from the same patient – we call this “registration” – so that we can look at the same position at different time points. In the case of lung cancer, adding tracking algorithms allows us to present the entire history of every nodule in a lung to the radiologists when they open a case. Instead of having to look up prior scans and navigate to the right position for a few example nodules, they see everything at once. This should not only free up time, but also make for a more pleasant working experience for the physicians.
Radiology will evolve because of AI. The question is, how?
There are several directions where AI is progressing rapidly. The obvious one is that we are collecting more diverse and representative data to build robust models that work well in clinical settings. This includes not only data from different types of scanners, but also data related to co-morbidities that make the detection of cancer more difficult.
Aside from data, there is a continual progress in developing novel ML methods to improve accuracy. For example, one major area of research is looking at how to disentangle biological variability from differences in image acquisition; another area is looking at how to transfer ML models to new domains. Multi-modality and predication represent two particularly exciting directions that also hint at how radiology might change over the next few years. In precision medicine, integrated diagnostics is a critical direction aiming at using data from radiology, laboratory medicine, pathology, and other diagnostic areas for treatment decisions. If these data are used together, they offer a lot more information to guide decisions than any one particular parameter alone. This is already standard practice, for instance, in tumour boards; ML will simply enter into the discussion moving forward. This begs the question: what should ML models do with all this integrated data from multiple sources? One thing we could do is try to predict future disease as well as an individual’s response to treatment. Together they hold a lot of power that we can exploit to create “what-if” predictions that can guide treatment decisions.
Trends for 2025: shaping efficiency, quality, and reimbursement
There are several factors driving AI in clinical practice. Two important aspects are efficiency and quality.
Efficiency
By allowing radiologists to concentrate on the crucial and challenging aspect of their work – integrating complex data – AI can help to increase efficiency. AI can support this by providing critical and relevant information at the point of care – e.g. quantitative values – or by automating a few tasks such as detection or segmentation of an anomaly. This has an interesting side effect: it not only enables the assessment of changes to be faster, but it also brings tasks such as pixel-by-pixel segmentation and volumetry of disease patterns from research to clinical practice. Manually segmenting large patterns is completely unfeasible in many circumstances, but automation renders this information accessible during routine care.
Quality
AI influences quality of work. By that we mean: becoming better at diagnosis, the recommendation of specific treatment, the earlier detection of disease, or the more accurate assessment of treatment response. These are benefits for each individual patient. At the moment, the relationship of these benefits with cost effectiveness on a system level is being evaluated to study and benchmark the health economics impact of the introduction of AI in radiology.
Reimbursement
AI adoption is no longer solely about efficiency; it is being recognised and rewarded for its tangible contributions to patient care and cost savings. Its inclusion in reimbursement schemes highlights this shift. While the benefits – such as reducing unnecessary procedures and accelerating treatment – seem straightforward in hindsight, the journey has been long. Now, with the first successful cases emerging, the transformative impact of AI is clear. By improving patient outcomes and optimizing healthcare processes, AI is reshaping the industry, with exciting developments on the horizon.
Shaping the future of medical imaging
Medical imaging is undergoing fundamental transformations. Precision medicine, integrated diagnostics, and novel molecular diagnostic technology are changing how treatment decisions are made in an increasingly complex landscape of therapy options. AI is a catalyst of this change, enabling physicians to integrate more characteristics captured by different modalities and link them to treatment responses.
Startups in the HealthTech field play a vital role in driving these advancements. Startups are agile in nature and can address industry gaps by leveraging cutting-edge technologies like AI to develop targeted solutions. Their innovations are critical in areas such as diagnostic tools, medical imaging, and workflow optimisation. Startups’ technologies can help reduce inefficiencies, enhance patient outcomes, and make healthcare delivery more accessible and effective.
That said, widespread adoption of AI tools will take time due to technical challenges, integration issues, and health economics concerns. One thing we can all do to accelerate the process is to be informed patients. Asking doctors about AI tools they might have tested or currently use can help integrate these innovations into practice, complementing professional expertise and knowledge. The market speaks to demand; by advocating for early, accurate detection and improved care, we can help shape the future of medical imaging.
For more startup news, check out the other articles on the website, and subscribe to the magazine for free. Listen to The Cereal Entrepreneur podcast for more interviews with entrepreneurs and big-hitters in the startup ecosystem.