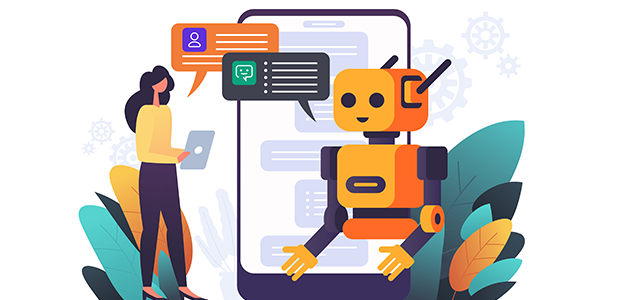
Natural language processing for business: a practical guide
ChatGPT is receiving an estimated 96 million visitors per month. According to Statista, in business applications the AI-powered chatbot is most commonly used by technical and educational organisations.
A strategic use of natural language processing tools in applications where human-like responses are helpful can improve productivity, by automating laborious tasks, reducing administrative time and freeing up employees for more productive tasks. Its ability to identify additional insights from data can also lead to better decision-making. However, businesses looking at implementing natural language processing tools have concerns about cost, privacy, bias, risk and impact on their workforce.
Here Alex Luketa, CTO at artificial intelligence (AI) for business consultant Xerini explains how businesses can get the most out of generative AI.
While it’s exciting to type a quick query into ChatGPT and read the results, the real value of AI will be realised when businesses can seamlessly integrate it with existing systems and data. Once connected to a company’s internal systems, AI can help solve challenging business problems in a quick and cost-effective way.
Applications of OpenAI in business
Some of the most impactful areas to use AI in business are in data management and analysis. Both structured and unstructured data can be tagged and classified, so that information is more accessible and easier to find using natural language search. For example, a business may want to onboard thousands of legacy certificates so that data is easily available to staff, or desire the ability to simply ask a system a question a client has posed, rather than spend hours manually searching for the answer. Another example would be for data analysis, such as automatically screening CVs to shortlist candidates for a job role. Many of these tasks would have been too labour intensive or technically challenging to be worthwhile. Before AI, the cost vs benefit didn’t stack up — now it does.
There are hundreds of artificial intelligence tools and models out there with varying use cases, which can make the market difficult to navigate. There is no universal tool for every application, and choosing the right tool is important, so before investing in a tool, a business needs clarity on its capabilities. This means achieving good visibility on the data it does — and doesn’t — collect, knowledge on where and how is stored, a clear articulation of the problem that needs to be solved, and the expected benefits of solving it. After establishing this, businesses can explore various options, including using OpenAI in its current state, enhancing its functionality through integration with a low-code data orchestration platform like Xefr, or opting for a fully customised private model.
Addressing the weaknesses of Open AI
In our experience, to gain a true competitive advantage, businesses will need to do more than just use standard models. However, this doesn’t have to be complex — software engineers can build a straightforward wrapper that transforms an OpenAI model into something specific to their use case. For example, wrapping GPT-style models with prompts or guard rails can help configure them quickly and help overcome accuracy issues. OpenAI tools can also be made more traceable, formatted to show why an answer was given with links to the source material so that humans can double check the answers.
Bias is another weakness to address in OpenAI systems. I experienced this for myself, when I recently asked ChatGPT to write a thank you note to my wife, and its answer heavily emphasised her role in the household and as a mother. Naturally, businesses do not want to implement biased AI systems, particularly if they are using the tool for something sensitive like screening a CV. Software consultants can help build guard rails and prime an OpenAI system to minimise biases or train an AI tool on the business’ proprietary data, which is less likely to contain biases.
Managing privacy concerns
ChatGPT is fuelled by online data, and employees may inadvertently hand over sensitive data in their queries — a privacy concern. It is possible to make small modifications that sit over the OpenAI API to improve this, such as to hold the data for a limited number of days, and ensure it is not used for training.
Often when engaging with a consultancy to develop bespoke solutions, businesses would prefer to retain ownership of IP. Often, it’s not the tool or the AI model (these often use open-source libraries and sometimes amount to a few lines of configuration code) that amount to IP but the data + model + training process giving rise to a trained system. The data, configuration and trained-model weights amount to the IP that will be unique for each client and is something that they can own. Where we do need to write custom software (e.g. business-specific calculations) then we can do this in auxiliary projects that are separate to Xerini codebases and this is something the customer (or partner) can retain IP ownership of.
Finally, where there are privacy concerns, we can train and fine-tune open-source models that are bespoke to the client and can run on private hardware without leaking data to third-party services. For example, if a company were handling private medical data and wanted absolute control of it.
Managing the process
Any investment in technology is ultimately a risk. In the current climate, the largest barrier is ensuring that the benefits are tangible and properly understood. Taking an incremental approach, and breaking things down into manageable phases that each deliver identifiable value but carry less risk can be a pragmatic approach.
There is also a people concern, especially with a fear of losing jobs or even agency within their current roles. This has always been true of technology but is more so with AI. For people-centric concerns, it’s important that we convey a message of enhancement rather than replacement. Employees will be able to get more done in less time, and this will make their lives easier rather than making their role redundant. Getting this message across is key because it reduces the number of objectors and potentially turns them into champions.
Working with an experienced software consultancy can streamline the AI integration process greatly. An AI expert can help identify which tools will best meet the needs of your business and can customise them to deliver more value. This can help avoid expensive platform migrations and mean you can integrate your desired AI functions quickly and smoothly.
We believe all businesses regardless of size and situation are ready to start their AI journey. Whether it is through making better use of available tools like ChatGPT, through integrating their systems and data via platforms such as Xefr or with fully bespoke model generation.