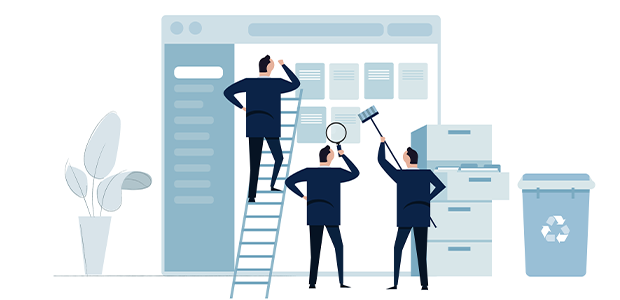
How Bad Data Poses a Multimillion-Pound Risk to Your Business
Every business contends with a level of 'bad data' - information marked by inconsistency, contradictions, and fragmentation across application silos.
Organisations that successfully minimise the impact of ‘bad data’ realise substantial rewards that enhance their bottom line, while those neglecting this issue face challenges that can result in multimillion-pound losses annually.
Gartner’s experts emphasise the severity of this problem, highlighting in an article on data quality that poor data quality costs organisations an average of $12.9 million each year. This not only contributes to financial losses but also amplifies the complexity of data ecosystems and leads to suboptimal decision-making.
In this context, we delve into the critical impact areas that SMEs and startups may encounter when they overlook the importance of data quality, examining the costs and missed opportunities, and what can be done to help avoid this significant financial risk.
Poor Business Decisions
Leaders at executive and financial levels rely heavily on data for strategic forecasting and analytics, forming the backbone of decision-making processes crucial for organisational growth. The examination of metrics such as cash flow, customer and product profitability, and sales effectiveness plays an instrumental role in shaping key business strategies.
Despite the advanced capabilities of modern analytics tools, their effectiveness is inherently tied to the quality of the data they process. A significant challenge arises when data discrepancies exist across core applications, leading to the management of multiple versions of the truth and decisions based on informed conjectures rather than concrete insights.
This concern is widespread, with a survey by Experian revealing that 38% of respondents believe poor data quality damages the reliability and trustworthiness of their analytics. Supporting this perspective, Melody Chien, Senior Director Analyst at Gartner, emphasises in an article entitled “How to Improve Your Data Quality” that “good quality data provides better leads, better understanding of customers and better customer relationships.”
Diminished Productivity
A common approach to readying data for business use involves manual reconciliation by staff. This process entails extracting data from source applications and addressing significant discrepancies through a spreadsheet or a similar tool.
Alternatively, in situations where customer service agents or account managers handle inquiries related to bills or licenses, inaccuracies or incomplete information within a single application can trigger a cascade of requests to colleagues across various channels to rectify the customer’s query.
These labour-intensive data tasks incur a substantial cost. The hours dedicated to cumbersome data preparation accumulate into sizable monthly expenses and contribute to diminished staff morale. Furthermore, these efforts divert precious time away from more valuable tasks crucial for business growth. It’s unsurprising, therefore, that the Experian survey mentioned earlier, identifies “wasted resources and additional costs” as the primary concern associated with poor data quality, acknowledged by 42% of respondents.
Manual data work is not sustainable in our on-demand digital age, especially for SMEs and startups. The limitations of error-prone manual work make automated master data management a compelling option to address data issues.
Subpar Customer Experiences
Customers today demand seamless interactions with businesses, favouring brands that not only comprehend their preferences but also anticipate their needs, delivering personalised experiences.
However, this ideal is jeopardised when customer data is siloed and inconsistent, irrespective of whether a business serves B2C, B2B markets, or both
The repercussions of bad customer data are manifold, including customer disappointment arising from products shipped to outdated addresses not updated in warehouse management systems, frustration due to customer service teams offering only partial views of order histories, and resentment towards email marketing campaigns providing discounts on recently purchased products at full price. Such discontent is amplified when shared online, leading to potential reputational damage and declines in Net Promoter Score (NPS) which could dissuade prospective investors.
According to Experian’s survey, 39% of respondents identify the impact of poor data quality on customer experiences as their second most significant concern, with 85% of respondents indicating that poor-quality customer contact data adversely affects operational processes and efficiency.
Achieving true customer centricity necessitates a comprehensive, 360-degree view of customers across all channels, providing a competitive advantage that not only delights customers, but also fosters trust, and cultivates long-term brand loyalty.
Sidestepping Data Land Mines
Allowing the proliferation of bad data introduces a host of additional risks, including but not limited to:
- The potential loss of revenue as cross-sell and upsell initiatives falter due to inaccurate data, leaving opportunities untapped.
- The risk of regulatory non-compliance, particularly when mission-critical data fails to align seamlessly within core systems.
- Sluggish and labour-intensive onboarding of new employees or partners can slow time to value and leave subpar first impressions.
- Acquisitions may fail to deliver quick and effective value as new data struggles to integrate seamlessly with existing information in your systems.
Build a Foundation For Success with Data Integration and Management
Addressing bad data is not just about avoiding financial losses; it's about fortifying the foundation upon which various business functions and strategic initiatives rely.
Effective data integration and management play a pivotal role in mitigating the financial risks associated with 'bad data' within a business. Inaccurate, inconsistent, or fragmented data can lead to costly errors in decision-making, impacting everything from financial forecasting to customer interactions.
By integrating and managing data systematically, organisations ensure a unified and accurate view of their information ecosystem. This not only enhances the reliability of business insights but also safeguards against regulatory non-compliance, potential revenue loss, and operational inefficiencies.
The ability to make informed, data-driven decisions hinges on the quality of integrated data. A well-structured data management strategy not only helps avoid financial pitfalls but also fosters a foundation for innovation, strategic growth, and sustained success in today's data-driven business landscape.