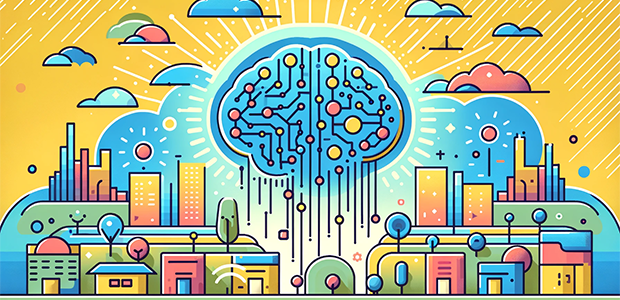
How AI Startups Can Get Enough Data and Avoid Hallucinations
All AI models are based on data – the core of their work. Without it, even the most robust algorithm is useless because it cannot learn. And for AI startups, this can be a real problem.
Tech giants like Google or Microsoft use massive amounts of data to train their AI models. But there are other sources of data: technology corporations can also access information from other companies in the market. Suppliers, partners, and customers are more willing to share their data with them due to the credibility of brands.
However, getting this access can be a challenge. Businesses keep their data private for many reasons – including confidentiality, regulations, and customer loyalty. This is especially true for sensitive information – for example, in areas such as finance or healthcare. Finally, access to exclusive data can be a competitive advantage that prevents companies from sharing it with AI platforms.
Getting new data can be challenging even for corporations: researchers warn that high-quality natural data could run out in 2026, while low-quality text and image data sources will be exhausted between 2030 and 2060.
But for startups, getting access to data is a real problem. Without their own data stream and partners ready to share the information, they must train their models on open sources, which is often insufficient to build a powerful service. Among other things, this leads to hallucinations in the system operation.
“Eager-to-please intern”: hallucination problem
The lack of comprehensive training data is one of the main reasons for hallucinations in AI systems. If the information is insufficient or contains essential gaps, the model will not see the full picture and may produce false outputs.
What do AI hallucinations mean? In some cases, the AI gives false information or non-existent facts that aren't connected to reality. This term is usually used in the context of large language models (LLM) or computer vision systems, and this is still a big problem for market leaders. Hallucinations are produced by ChatGPT, Google’s Bard, Midjourney, DALL-E, and other large systems. You can find many articles on the Internet, starting with “We asked ChatGPT about…” that talk about how the neural network gives incorrect answers and invents non-existing facts. It’s a reason why Professor Ethan Mollick of Wharton has called ChatGPT an “omniscient, eager-to-please intern who sometimes lies to you.”
Because of hallucinations, using AI systems in business is risky. There is a good example: recently, it was revealed that a lawyer, Steven Schwartz, used fictitious cases created by ChatGPT in the trial. Just imagine what would happen if AI tools were allowed in judicial practices.
Collaboration problem
As we see, hallucinations are inevitable at the current level of AI model development, even for the biggest of them. But for startups with limited access to data, algorithm distortions can be critical to their entire functioning. A designer would instead choose Midjourney or DALL-E over some unknown AI. Otherwise, he risks his character having three arms or floating in the air.
What can startups do to get access to data? Collaborating with large technology companies with their datasets is the most obvious way. According to the 2020 Social Science Research Network research, nearly 50% of respondent firms already partner with large high-technology firms to access data. Some startups collaborate with big enterprises like EY. But although now EY has startups that the firm provides with its data, some executives are concerned about what might happen to their information if it is used for an external AI model.
Another example is Uber, which recently open-sourced its dataset for autonomous driving research, so developers worldwide can access it.
Here, we run into a problem I already mentioned: companies don’t willingly hand over their data to startups. In other cases, they ask a high price for access to it. This happened with the AI startup Vessel, which allows people to “try on” clothes virtually online. To improve the model, the company tried to convince some large retailers to share their data, but in return, it was asked for huge payouts and even equity in the company.
Searching for solutions
Another option for AI startups is to partner with a company with a lot of comprehensive data but lacks AI expertise. For example, develop a model for medical diagnostics. It may be wise to build a collaboration with a network of medical centres so you can gain access to its data. But there are some risks that you will have to sell majority control of the company.
Also, startups can train AI models for each client using only that client’s data. But often, it’s not enough for high-quality system operation. Moreover, clients do not always agree to that quickly: they want to be confident in your cybersecurity expertise and ability to protect their information.
Finally, there is an option to train AI models on synthetic data — a computer algorithm generates this data type and imitates natural data. However, unnatural data can be imprecise and unreliable, leading to hallucinations or bias.
Of course, there are more ways for AI companies to gain data. The market is growing incredibly fast, and startups should focus on finding a reliable source of comprehensive, relevant data, giving them a significant advantage over competitors.