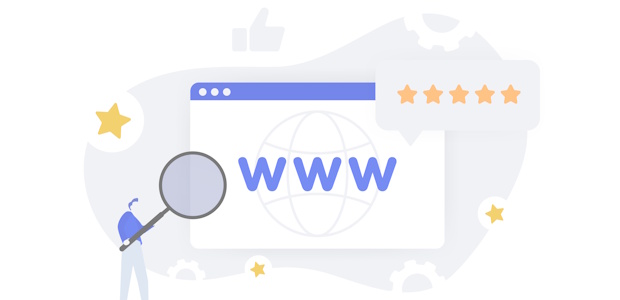
The secret weapon startups have over big tech in the AI race
The AI revolution is often depicted as a contest between tech giants, each pouring billions into ever-larger, general-purpose models. But while big tech dominates headlines, a quieter transformation is underway: startups with deep domain expertise are building deeply valuable, practical AI solutions that solve real problems. In a world obsessed with big names, it’s these focused, specialist teams that are quietly reshaping industries from the ground up.
Why domain expertise is the next frontier
The limits of general-purpose AI are becoming clear. As leading voices in the field have pointed out, models trained on all the world’s public data can only go so far before they hit a ‘data wall’. The next leap forward will come not from simply scaling up, but from embedding AI with the context, nuance, and workflows of specific industries. In reality, domain expertise is the next frontier of AI.
This is especially true in sectors like law, healthcare, and finance, where accuracy, compliance, and trust are paramount. In legal tech, for example, using proprietary internal data and expert-written context can dramatically improve the quality of LLM output. The result isn’t just more accurate, but it also genuinely fits the needs and realities of professionals and their clients.
Building with constraints: how scarcity fuels innovation
New entrants like DeepSeek have shown us that constraints drive innovation, and they aren’t the first to teach us this lesson. Take the story of the mini-mill in the steel industry: dismissed by incumbents as too limited, these smaller players used new technology to serve overlooked market segments. Over time, their focus and efficiency allowed them to move upmarket and disrupt the entire sector.
I’ve learned firsthand that innovation can thrive in resource-constrained startups that are forced to prioritise ruthlessly. When you need to deliver tangible value from day one, rather than chasing hype or vanity metrics, you naturally lean into agile, iterative development cycles-testing early, learning fast, and pivoting boldly when needed. This kind of working methodology makes it much easier to adopt new transformative technologies like GenAI that larger organisations often struggle to match.
Turning industry knowledge into AI advantage
Domain expertise is not just a ‘nice to have’ – it’s a strategic moat. Teams with deep customer and domain insight bring an understanding of pain points, regulatory requirements, and user behaviours that generic AI cannot replicate. This knowledge shapes everything from data collection and model training to user experience and product-market fit.
You can leverage this advantage in your startup by:
- Partnering closely with industry insiders and end users to surface real-world problems
- Curating proprietary datasets that reflect the subtleties of your domain
- Embedding subject-matter expertise into every stage of development, from prompt engineering to risk assessment
As AI continues to unbundle professional services, the winners will be those who can translate deep knowledge into targeted, trustworthy solutions. This is already happening in legal tech, where vertical-specific AI is enabling the ‘great unbundling’ of services-making expertise accessible at scale, at a fraction of the cost.
Practical guide: implementing AI with minimal investment
When adopting a brand new technology like GenAI, the key is to think small and focused. We began by identifying a single, high-impact use case where AI could deliver measurable value, and building from there. We involved end users early and often, and weren’t afraid to ship imperfect versions, as feedback is the best teacher.
Internally, AI can streamline operations, automate repetitive tasks, and free up your team for higher-value work. Externally, it can power new features, enhance customer experience, or even create entirely new business models. The secret is to stay laser-focused on the problems that matter most to your users, rather than getting distracted by the latest AI trend.
Responsible AI: managing risk with sensitive data
If you’re working in industries with sensitive data, especially in fields like law or healthcare, it may feel like GenAI is too new and risky, but there are safeguards that you can put in place:
- Use platforms like Amazon Bedrock that provide LLMs within your own company’s infrastructure
- Build data and privacy risk assessments into your product iterations, making sure each experiment has mitigations built in from the beginning
- Being transparent with users about how AI is used and how decisions are made
Frameworks such as the NIST AI Risk Management Framework and guidance from organisations like ITechLaw offer practical blueprints for responsible AI adoption. Building trust is not just an ethical imperative-it’s a competitive advantage.
The age of the specialist
The future of AI belongs to the specialists. As big tech chases general intelligence, startups with deep domain expertise are quietly building the next generation of transformative, trustworthy AI solutions. For founders, the lesson is clear: embrace your constraints, leverage your unique knowledge, and focus relentlessly on real user needs. In the race to unlock AI’s full potential, it’s the depth of understanding-not the size of your model-that will set you apart.