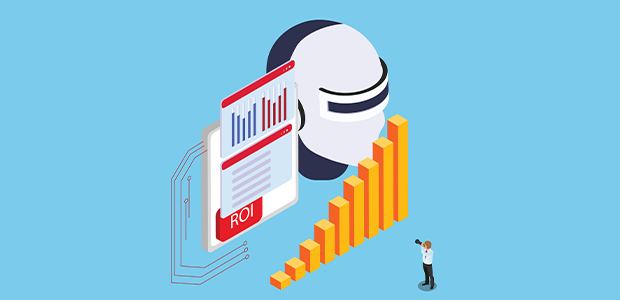
Four ways enterprises can improve their ROI on AI
When generative AI exploded into the public consciousness two years ago, business leaders were understandably keen to be at the vanguard of the revolution and unlock its potential.
This is reflected by enterprises investing heavily in AI technology. This year, the global AI market was predicted to be worth $235 billion, rising to $631 billion by 2028, according to an IDC report.
However, many enterprise investments in AI are falling short of expectations and not achieving anticipated returns. Gartner recently reported up to 30% of GenAI projects will be abandoned by the end of next year, citing return on investment (ROI) as one of the issues.
This was reinforced by an Appen report, published last month, suggesting enterprise deployments of AI initiatives are down: from 56% in 2021 to 47% this year. It said the trend was “likely driven by diminishing ROI or the lack of significant outcomes, likely due to the increasing complexity of AI models and the challenging problems they aim to solve.”
Conversations around ROI on AI are becoming increasingly common with clients and I have seen a noticeable shift towards more rigorous investment decisions as the hype is replaced by a pragmatic focus on business results.
So if we start with three assumptions – 1) we’re still at the beginning of the AI journey; 2) AI use cases and capabilities are evolving quickly; and 3) AI will play an important role in the way we create business value – then the question we need to address is…
How can businesses improve their ROI on AI?
It should be pointed out there isn’t a one-size-fits-all approach when measuring AI ROI. Each business has unique needs and objectives so measurements must be tailored to align with an organisation’s specific goals. However, there are four actions that can be considered and/or adapted for improving ROI on AI.
1. Rethink the ROI metrics being used
Most established organisations have defined ROI criteria, particularly for technology investments. However, AI is more than a traditional tech investment – it’s a transformative business tool, and so its impact should be measured differently.
Investing in AI is less of a technology upgrade and more of a leap: like moving from aeroplanes to teleportation. Therefore, the way ROI is measured needs to evolve so it can gauge both immediate gains and long-term potential for exponential growth and disruptive opportunities.
To effectively assess AI ROI, enterprises should categorise and prioritise projects by impact, cost and complexity… and identify those that deliver quick wins, competitive advantage or disruptive industry innovation. For each category, fit-for-purpose quantitative and qualitative metrics should be established.
Given the scale of AI investment needed to enable a business, senior leaders must adopt a strategic, long-term perspective, often looking over the horizon and with a payback period extended beyond what they would typically apply.
2. AI should be business-led
Machine learning, neural networks, multimodal, AGI, LLMs… as with most technologies, the amount of acronyms and buzzwords thrown around is almost comical – and this can make the topic of AI feel impenetrable. As a consequence, business leaders often cede leadership on AI transformations to their tech and data teams.
However, AI is fundamentally a business tool and should be business-led. It is the business leaders who have the holistic view of their organisation, their market and what the overarching strategy is.
Leaders therefore need to empower themselves with knowledge and skills. This will enable smarter investment decisions and align these more closely with strategic outcomes.
3. Choose the right projects
Selecting the right AI projects is crucial to driving both short and long-term ROI. It may sound obvious but to date, many enterprise deployments have focused on experimentation and learning, often in isolated silos and without clear alignment to a broader AI strategy.
Developing a cohesive AI strategy enables the organisation to connect AI investments directly to business goals. It also allows for categorising and prioritising projects with the appropriate quantitative and qualitative metrics, as touched on above, ensuring resources are objectively allocated to initiatives with the highest potential impact.
4. Build the data foundations
No data, no AI. It’s almost that simple.
According to Forrester: “data quality is now the primary factor limiting GenAI adoption.”
Data is a critical asset for AI. High-quality, consistent data improves model performance and leads to more accurate insights. Conversely, poor data quality, such as inconsistencies or incomplete records, can lead to inaccurate predictions and wasted investments.
Establishing a robust data governance framework is equally important. This should clarify ownership, usage, and ensure compliance with regulations. Strong data governance builds trust and allows data to be used across departments without extensive rework, making it easier to scale AI projects.
Investing in data quality and governance is a strategic move that will reduce risks and maximise the value of AI.
We are still in the early stages of the AI journey, but it's moving fast. AI is poised to transform entire industry sectors and give rise to new business models that we are only just beginning to imagine. To achieve the greatest long-term ROI, enterprises must focus on building the right AI foundations today. This strategic investment will position them to move faster and capitalise on new opportunities as AI continues to evolve.
For more startup news, check out the other articles on the website, and subscribe to the magazine for free. Listen to The Cereal Entrepreneur podcast for more interviews with entrepreneurs and big-hitters in the startup ecosystem.