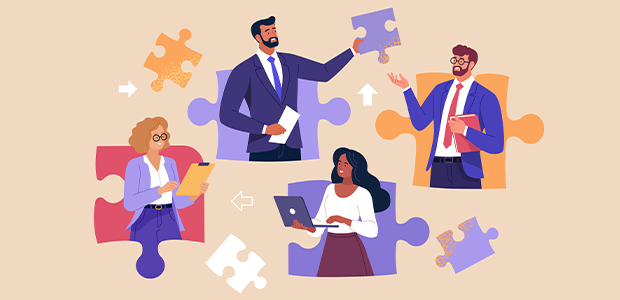
Don’t augment employees with AI, augment how well employees work together
When money is cheap, we want AI to be more human, or even more than human. Capitalists may even secretly dream of replacing their meddlesome employees with bots while claiming otherwise.
But when the cash spigot closes, as it appears to be today, we lose interest in AI parlour tricks. Leaders just want our smaller, overworked teams to be able to keep up, as a team, before they burn out and we go under. A chart every executive should have shows the cost of labour relative to productivity. If your cost of labour goes up as productivity goes up, you’re in trouble right now.
We’re all going to die
Population growth is slowing and so is productivity.
America is on its way to being 86,000 physicians short of demand by 2036. Everyone from hospitals to urgent care facilities and imaging centres face a staffing crisis. Demand for pathology services for instance far exceeds available pathologists, risking costly delays.
We enjoyed the highest rate of new pathologists joining the workforce in the 1960s through 1980s. After that, a team led by Dr Stanley Robboy of Duke University points out the number of retirees exceeded the number of new pathologists. Their numbers show since 2000 we’ve had 600 more pathologists retiring each year than new ones stepping in.
It’s not just talent shortages. It’s what we’re asking talent to do and the sneaking suspicion that technology is not keeping up, or is even slowing us down while adding errors as it goes along.
Dr Robboy points out in 1996 a pathologist examined a breast lumpectomy by putting one slide with tissue under a light microscope to decide if there was cancer, was it invasive and determine the margin. Now the pathologist looks at 40 to 50 or more slides. Added views and steps include stains and molecular or proteomic tests.
Dr Donald Karcher of George Washington University says in The Dark Report digital pathology isn’t keeping up – “it will help workflow to a certain extent. But when you ask pathologists who use digital pathology on a regular basis, they will tell you it takes them more time to sign-out cases.”
So it’s not a surprise that healthcare talent is the main factor shaping business goals for 36% of organisations in that industry, according to Gartner. That’s more than any other variable including supply chains or competition.
The story is the same in accounting, manufacturing, insurance, and really every sector. Fewer accountants, skilled laborers, claims adjusters and underwriters is correlating with more accounting errors, product defects, claims mistakes and poorly assessed risk.
And when invited to help, AI may not only be failing to increase productivity, but it may also be further reducing quality at the same time. A pilot study by the University of Massachusetts of 100 clinical summaries from generative AI showed AI producing longer summaries and making bold, two-step reasoning statements, leading to hallucinations. Some generative AI in the study hallucinated less by avoiding extensive inferences, but those summaries were of lower quality.
No wonder 60% of Americans told the Pew survey they would be uncomfortable with medical providers relying on AI to deliver healthcare.
So what’s wrong? Too many leaders are asking AI to do the wrong things, and failing to understand what it needs to help do the right things.
Rediscovering workflows
Business or any endeavour at a big enough scale to matter is a team sport. Workflows are how the individuals on the team play as a unit. Discoveries or insights – AI generated or otherwise – are no good if a team can’t execute on them at scale. As the Mark Zuckerberg character says in The Social Network about the value of insight alone, “if you had invented Facebook, you would have invented Facebook.”
Execution “at scale” can only be achieved when a team achieves more than the sum of its parts. How much more than the sum is determined by what happens in the sequence of handoffs and coherence in the spaces between the individuals, the passing game.
Maybe that’s not new, in 1992 Steve Jobs was pointing to former Pentagon Chief Information Officer Paul Strassman’s analysis showing companies who invested in technology for team productivity outperformed companies that invested in tech for manager or individual productivity. But we seem to keep forgetting that lesson. And easy money makes the forgetting easier.
How do we get from here to there?
First, if you’re looking at how technology makes individuals more productive, as you can see, you need to stop. Your colleagues in business and investors have stopped doing that at an increasing rate and as money dries up for all the reasons I described above. They and you can’t afford it.
Second, if the problem is workflows, and that’s something that happens between individuals, communications is one way of describing that. Slack’s Stuart Butterfield has claimed 50-60% of the average employee’s time is spent communicating.
If you abstract that across all workflows you see the problem you need to solve.
Consider the term EGFR in medicine. It can stand for “epidermal growth factor receptor” (a gene) or it can mean “estimated glomerular filtration rate,” (a kidney test). Two physicians that work together can infer which EGFR was meant by the other, but once you make the team bigger and bigger, and they have less direct communications with context, workflows go off the rails in this and many other ways.
We already see research showing that “democratising” data without addressing the clarity and variance in meaning is leading to slower work processes in healthcare and elsewhere. It’s not just entering the data - the most common complaint among physicians in my area of work - but also trying to understand what colleagues are telling you, or the interpretation of colleagues that computers spit out at you. That produces cognitive burden, and that cognitive burden is the physician trying not to make a mistake.
We need to prioritise solutions that can avoid that trouble, boost productivity, and cut costs. But they require an upfront investment by leaders in understanding how their teams work, and specifically how teams communicate the kind of information specific to that domain.
Very practical magic
If we want to get technical, and we’re going to need to get technical, let’s take neuro-symbolic AI designed to eliminate hallucinations, optimise explainability, and, crucially, inject reasoning into the AI pipeline using brain-like neural networks.
In addition to recognising patterns like regular generative AI, the goal is to comprehend abstractions and learn from the AI’s own mistakes. It looks at individual patient records, in my industry, to understand individual patient journeys and outcomes rather than drawing broad assumptions. That takes a lot of upfront work. But the work is necessary because we don’t really have a choice if we want to succeed.
Systems using this have been shown to outperform retrieval-augmented generation (RAG) and LLM-only techniques. In a recent study on the identification of patient cohorts from unstructured and structured EMRs, for instance, this way of doing things outperformed generative AI in multiple benchmarks, including precision and recall. Unlike LLM-only solutions, this approach also maintains a fixed cost per query, regardless of the database size.
And that, right there, gets back to our executive’s chart. If we can cut down the mistakes, the cognitive burden those potential mistakes incur as a team works together. And if we use the right domain expertise in our models, teams can get more efficient in their workflows, and they do. The technology actually becomes less expensive as well. It’s been documented as going down to pennies per query rather than dollars or hundreds of dollars.
Our executive’s chart then shows talent able to do more meaningful, accurate and scaled work without burning out. The cost curve flattens to get us out of our downward spiral.
Maybe workflows between all of us everyday employees seems boring compared to harnessing AI to help a genius individual discover a better treatment for cancer. But workflows that deliver the right care to the right patient, and that is accessible to more of us is the better treatment for cancer. And we can get the cures too for that matter.
For more startup news, check out the other articles on the website, and subscribe to the magazine for free. Listen to The Cereal Entrepreneur podcast for more interviews with entrepreneurs and big-hitters in the startup ecosystem.