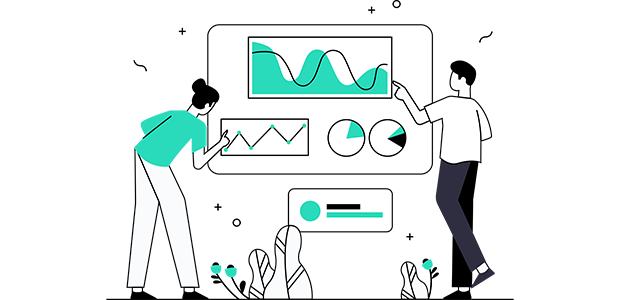
5 tips for building a data strategy to maximise startup value
A growing number of PE firms are using advanced data analytics techniques to assess their potential investments. As a result, they require more complex data from their prospects to build a much more holistic picture of how a company is performing now, and, with predictive analytics, will potentially fare in the future.
Everything from customer service data, product revenue, transaction levels, retention rates, organic versus inorganic growth, ARR, to customer profiles and team performance metrics are all of serious interest to investors.
In a nutshell, PE firms have gone beyond looking at the ‘what’ of the performance, to expecting management teams to be able to have the data to answer the ‘why’ as well. For startups looking to attract investors and maximise their value there is a clear incentive to be readily able to answer these questions. Being able to do so will help them control the investment conversation.
To achieve this businesses need to have a clear handle on all their data. They need to be able to analyse and report it in such a way that they can tell the most compelling narrative about their business. At the same time, one of the largest new cost outlays for many businesses is the implementation of AI tools and platforms. Both of these goals are interlinked. Each requires the right data infrastructure, expertise and procedures to be effective. Companies that are able to leverage AI to exact efficiency, creative, or productivity gains will, generally speaking, be much more attractive to investors. Outside of AI, reliable data analysis should be the bedrock of how a startup develops its offering and makes commercial decisions. Good data insights will lead to better decision making which, over the long term, will lead to higher growth and profitability, which again impacts a startup’s exit prospects.
So no matter how you look at it, the value of your startup to investors will be significantly influenced by how effectively you use your data. This in turn is largely predicated on the quality of your data strategy. As a rule, the earlier you create and implement a comprehensive strategy in your startup’s journey the more effective it will be. While there is no definitive right or wrong way to go about designing and executing it, there are some common pitfalls that, if successfully navigated, will give you the best possible chance of success.
1. Data for data’s sake
Too often, people start with the data, not the value creation plan and what they are ultimately trying to achieve as a business. This is the surest way to spend too much money and get limited results. Without a definitive objective, data and AI transformation projects can suffer from mission creep, cost, and time overruns and missed opportunities, and the objective must be about the business not about, for example, simply having clean data. Within your overall mission have a set of goals for your data work which are strategic, well-scoped, measurable, and actionable. For example, if you are looking to grow revenue from loyal customers, then you may want to stop customers from churning, and so you would want to then understand which customers are at risk of churn, and what are the drivers. This will then make it clear what tools, processes and expertise will help you on the way to achieve that goal.
This may all sound very simple, but, according to Harvard Business Review, the failure rate of AI projects is as high as 80%. A leading cause is an absence of defined objectives that align with an organisation's overall commercial strategy. Too many organisations see the adoption of AI and data analysis tools across their business as an objective in of itself rather than a means to facilitate a specific improvement. In short, your strategy starts with your objectives.
2. Ignoring the ‘boring’ tech
Investing in the right platforms to collect, manage, and analyse data is absolutely fundamental. This tech infrastructure must be accessible to every team and integrate into all your existing platforms in order to avoid the siloing of data. Existing platforms may be as simple as excel spreadsheets, so you want a simple data cube that can bring all this together. For many business leaders the nitty gritty of data management can feel, dare I say it, boring compared to the exciting potential of AI. As a result, it can be ignored or suffer from underinvestment. Yet, without these building blocks in place nothing meaningful can follow. It is critical to take your time to understand exactly what state your data infrastructure is in and which available technology platforms will meet your requirements now, and in the future. Conducting a data audit against the goals identified above should be the next step in any meaningful data strategy.
When you know what you need you can start the work of determining how to acquire and integrate these platforms, what skills you need to effectively operate them and what policies and processes need to be designed. These insights will help to further define your strategy. Often this doesn’t have to be a big technology investment, and something quite simple but scalable can set you up well.
3. Lack of integration into the business
Data and AI isn’t just about technology – it’s a cultural shift that requires company-wide engagement. If you build a set of dashboards which no one uses in their role there is no point in making this investment. This means you need to ensure you take the team on the journey, engage the stakeholders along the way and ensure the data work you are doing or the AI tools you are introducing solve their problems. You need to ensure you upskill the team to ensure they can interpret the core business data and use it to make decisions. Finally, you need to make sure you are meeting people where they are at. The best data-driven insights are integrated into how people do business, e.g. a cross-sell tool showing up in the chosen CRM that the sales people use every day recommending who they should contact in what order to talk to about which product on that specific day. This will get far better traction and results than some separate system that people need to log in to.
To harness the benefit of data for investment, you need to be able to use it to back up the investment thesis and story you are telling. Ensuring it clearly aligns, and your team is familiar with interpreting and talking about the data will put you in a much stronger position for conversations with investors.
4. Missing guardrails
As with all exciting disruptions, the increased reward is mirrored by increased risk; companies should be simultaneously assessing the commercial opportunities to leverage AI, as well as the risks it poses such as data privacy breaches and algorithmic bias. Across industries, we've seen that poorly governed AI can backfire, leading to legal liabilities, regulatory scrutiny, and reputational fallout.
Robust oversight is essential, beginning with clear guidance that outlines the purpose of AI adoption and explicitly defines what is – and is not – okay. This should be reinforced by practical safeguards and defined procedures so that employees understand their responsibilities, can identify irregularities, and know who is accountable for taking corrective action. Rather than being left as an afterthought, getting these structures in place should be established as a strategic priority from the outset of any AI initiative to ensure alignment, compliance, and long-term value protection.
5. Thinking short-term
It’s also important to note that becoming data-driven is not a once-and-done exercise. The technological landscape is continually shifting in new and often unexpected directions, bringing both opportunities and challenges. Knowledge can be easily lost or become obsolete. Governing policies and working procedures can become rapidly outdated.
To fully leverage AI and data analysis techniques, businesses must embrace a culture of continuous learning and improvement. Holding annual or, ideally, bi-annual training sessions for your team helps sustain this mindset. Additionally, conducting regular audits and policy reviews ensures alignment with best practices and emerging risks.
Think of becoming data-driven as an ongoing journey. Start by allowing employees to experiment with small pilot projects to evaluate their impact. Set clear KPIs for each initiative and conduct frequent reviews. Even on a small scale, these projects will provide valuable insights that can inform larger efforts. To support your progress, it's always advisable to consider partnering with a specialist data consultancy with experience that can help support your goals.
For more startup news, check out the other articles on the website, and subscribe to the magazine for free. Listen to The Cereal Entrepreneur podcast for more interviews with entrepreneurs and big-hitters in the startup ecosystem.