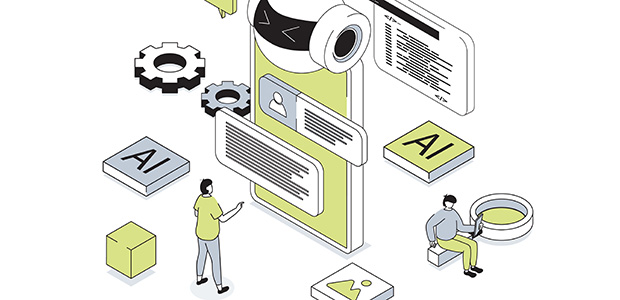
The next wave of AI innovation – a view from the trenches
As new governments are elected in the US, UK, and France – three countries that are among the world’s most prolific producers of AI startups – it is time for general thinking about artificial intelligence to shift dramatically.
To many of us in the trenches of AI development, it has become increasingly clear that there is a growing divide between what governments and regulators think is the cutting edge of artificial intelligence – large-scale generalist models that can exclusively be built by tech giants, which governments define as “frontier AI” – and the more focused models that those in the industry themselves consider to be the future of the majority of AI companies.
So, beyond the hype, what does the next wave of AI innovation look like?
Before the hype
In the wake of the release of ChatGPT in November 2022, social media was awash with conversations generated by Large Language Models (LLMs) and images generated by text-to-image models such as Dall-E, Midjourney, and Stable Diffusion. What this did very effectively was wake up the world to the potential of AI.
LLMs had been made possible by the introduction of a technology called transformers in 2017. Soon after, virtually all major tech companies started building towards LLMs – from Google’s BERT and T5, Microsoft’s Turing NLG, to models by Facebook, Alexa, and large Chinese corporates such as Huawei, Baidu, and Alibaba. The road to LLMs was being walked simultaneously by tech giants across the world.
And yet, I would expect very few outside the field of AI development to have any intimate knowledge of the above models. It was ultimately OpenAI (with access to very significant resources due to its unusual structure and mission) that ended up introducing the concept of LLMs to the general public, by adding conversational data into its GPT models. As I have mentioned in a Startups Magazine article previously, the sudden popularity of ChatGPT surprised even its own creators.
Other tech giants, however, swiftly came out with their own models, based on the predecessors mentioned above, and so the race – seemingly – was on. In the meantime, governments scrambled and saw the onslaught of LLMs and other large-scale generalist AI – all released in short succession after ChatGPT – as the new normal, frantically seeking to respond and quickly beginning to draft AI regulation on the assumption that this was what the future would look like.
After the hype
Recently, large scale models are moving away from being exclusively language-based, now able to deal with different types of input simultaneously (termed “multi-modal”), which brings us to what we call “foundation” models, of which LLMs are considered a subset.
As most of those in the trenches of AI development would agree, however, the future of artificial intelligence is very unlikely to be hundreds of companies producing very large-scale generalist models of the type discussed above.
These models require vast resources to train and expand – with vast data and computing requirements translating into huge costs. Famously, OpenAI reported a $540 million loss in the same year it released ChatGPT.
And while it is true that large scale models get more effective all the time, long-term profitability and competitiveness will require that those at the forefront of designing such models also keep growing capabilities commensurately with increases in efficiency, meaning cost savings will struggle to catch up.
Of the generalist large-scale LLMs currently in play, then, most of us believe only a handful – perhaps three or four – will ultimately come to dominate, with little need for others in the market. For specific tasks, and on the edge, models that are smaller, more efficient and use fewer resources will generally be used (as seen in the rise of Small Language Models or SLMs, developed by Microsoft, Meta, Google, and Apple).
The future of AI
That does not mean that other very large-scale foundation models will not continue to be built and built upon. The future of AI relies on, condenses, modifies, and learns from large generalist models – smaller focused models such as SLMs, for example, would not be possible without having developed LLMs in the first place.
It does mean, however, that world governments looking at the current competition and multitude of very large-scale generalist models – and deciding, by defining only such models as “frontier”, to ignore everything else – are essentially extrapolating from a temporary landscape. The current landscape is very much the result of a development process, and filtering in this way will exclude the majority of the future AI economy.
Most companies in the AI space, and no doubt many of the most profitable AI companies of the near future, will deploy cost-effective focused models that at present do not satisfy governments’ definitions of “frontier AI”. And yet they will very much represent the cutting-edge of applied artificial intelligence.
In planning for the future, then, if we do not widen our scope when designing legislation that seeks to establish a well-understood and well-regulated playing field for AI, we are setting ourselves up for failure – unable to anticipate the needs of a mature AI economy.
At the risk of making a ham-fisted analogy, after the invention of the proverbial combustion engine, we should not just be stockpiling materials for ever larger engines; we should anticipate the emergence of planes, cars and factories, and all the technologies our new engines unlock.
This article originally appeared in the July/August issue of Startups Magazine. Click here to subscribe