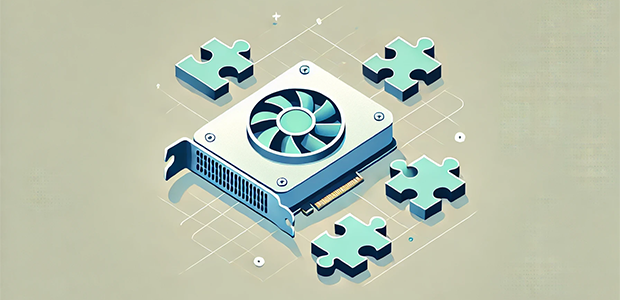
How AI startups can solve the GPU pricing puzzle – 6 tips from a CFO
For AI startups, choosing a GPU provider is one of the most important decisions they will make. The right provider can be the key to explosive growth, while the wrong one can be a massive drain on already limited cash reserves.
Generative AI startups are spending up to 70% of their budget on compute, so even saving a few percent on the right cloud provider can have serious implications for long term viability. Here are six rules to help you find the right GPU provider.
Pick the perfect partner
GPU options range from the so called “bare metal” clouds all the way up to the big hyperscale cloud providers like Oracle, AAWS, Microsoft Azure, and Google Cloud.
“Bare metal” GPU providers might look like the most appealing option from a pricing standpoint, but let’s investigate this a little further. Firstly, they offer direct access to dedicated servers without a virtualisation layer – which means they take care of the hardware, but you deploy the software stack on your own. While they offer the lowest compute pricing, they require a high level of additional technical expertise from your team and this additional expenditure on headcount and the associated costs or hiring in professional DevOps services can eventually add up to kill your savings.
At the other end of things, hyperscalers do offer a high level of service and flexibility, but they also come at the highest price on the market. If you have very deep pockets or sufficient scale of model, they’re a great option; but for startups, these costs might not be justifiable, based on your needs. You can however try to check their special programmes for startups, especially at early stages, to see if they are the right fit.
One compromise – sat right in the middle of these two options – is GPU clouds, like Coreweave, Lambda Labs, or Nebius. For startups, these providers offer the best balance between cost, services, and performance.
Go beyond the price tag
Many cloud providers list GPU prices on their websites, but don’t include costs for vCPU, RAM and storage – as well as the additional data transfer costs. Advertising pricing like this helps to make prices more attractive, but all these extras will add up. When it comes to comparing providers, make sure you compare like for like and calculate the total cost of the infrastructure you need with any provider you are considering – rather than just the standalone GPU costs.
Beware the hidden costs
The lowest price of any product is always subject to some special conditions, and GPU hours are no exception. The cheapest listed price of GPU often comes with a long commitment that must be paid in advance. Not only does this require a large amount of capital to foot an up-front payment, but it also limits your flexibility to switch providers or redeploy your capital further down the line.
Test before you invest
If you are training AI models at scale, before making any long-term commitments, it’s important to conduct a proof-of-concept test. Every cloud provider is different and the best way to ensure they can meet your technical requirements is to conduct smaller test trainings to assess their hardware’s speed and performance. A more expensive platform that performs better may work out cheaper in the long run, as although you pay more per GPU hour, you require fewer hours to train your models.
While not every cloud provider publicly offers a free trial, for a large enough contract, many will be open to some kind of proof-of-concept period - after which you can renegotiate your contract length.
Demand top-tier support
The level of support available will always depend on the amount of compute you are purchasing – and this is a rule for all providers. If you are only training a model on a single GPU, you can’t have the same support expectations as a customer who has 512 GPUs being used in production.
Therefore, when you’re choosing between cloud providers, consider the level of support each offers. If you are planning to train large models or use a cloud provider for resource-intensive inference, you want a dedicated support engineer and an SLA that guarantees 24/7 support. Anything less could create problems in the future.
Optimise your spending
The right provider and plan depend entirely on your unique needs as a startup. You will more often get better prices if you are prepared to pay up front to reserve some dedicated quantity of compute; but it may not be optimal for your needs – and it often requires a large amount of cash.
A pay as you go (PAYG) plan will cost significantly more per GPU hour, but the flexibility to scale up and down your usage as needed may be worth it. Not all workloads require the kind of 24/7 GPU power that the cheapest plans are designed for. Some providers allow you to combine the two options: so, you reserve your minimum GPU requirements and pay an additional fee for any usage above that.
The best option is to do the maths and model out the full costs of different scenarios to see which provider can give your startup the best balance between price, plan flexibility, and, of course, performance. Sometimes, the best hour an ML team can spend in a day isn’t on training an AI model – but optimising the compute costs.