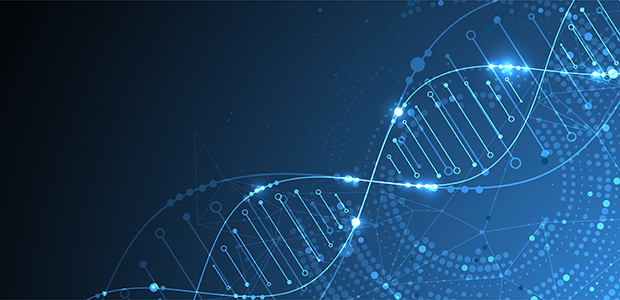
A ChatGPT-like tool that designs enzymes
Basecamp Research in partnership with the Ferruz Laboratory at the Institute of Molecular Biology of Barcelona announced the release of ZymCTRL (‘enzyme control’), a ChatGPT-like tool that generates new sequences from scratch based on a user simply typing in an enzyme identification code, which specifies the desired activity.
Large language models (LLMs), such as ChatGPT, have proven useful in helping scientists design and generate protein sequences. However, current models require further training as well as conditioning on a known protein starter sequence (‘seed sequence’) for protein generation.
ZymCTRL is a next-generation end-to-end protein LLM that offers rapid, cost-effective design capabilities for generating artificial enzymes. In contrast to other LLMs, the tool requires no seed sequence, giving end users complete control. Another important feature is ZymCTRL’s ability to create enzyme sequences that work but share only 30% resemblance to those in the training set – expanding the possibilities for designing new enzymes.
“With ZymCtrl, generating highly specific enzymes is as easy as interacting with a chatbot,” said Noelia Ferruz who has been partnering with Basecamp Research for over two years. The Ferruz lab is considered a pioneer in the field of AI for protein design, having previously built ProtGPT2. a deep unsupervised language model for protein design.
“Even before the release of ChatGPT, we began working on large language models with Noelia because we think these models represent the future of biological research and protein design,” said Dr. Philipp Lorenz, CTO of Basecamp Research. “We’re deeply excited by these results and ZymCTRL’s ability to create functional enzymes that can solve some of today’s biggest challenges, from finding new ways to treat devastating diseases to building greener and more sustainable catalytic processes in bioindustry.”
The open source ZymCTRL model has been independently reviewed by academics in Structural Biology and ChemBioChem, peer-reviewed scientific journals. In ChemBioChem, researchers at The Institute of Biochemistry at Austria’s Graz University of Technology, cited ZymCTRL’s efficiency and ease of use. “ZymCtrl designs putative enzyme variants on consumer GPUs within seconds and, remarkably, it creates these sequences with only an EC number as input,” wrote Horst Lechner, principle investigator for the institute, which is focuses on enzyme design that differs from what’s seen in nature.
Basecamp Research is sharing ZymCTRL open source with researchers and sees an array of potential applications, including designing enzymes for disease treatment and diagnostics, biofuel production, sustainable agriculture innovations and much more.
While ZymCTRL was initially trained on publicly available datasets, it can also be integrated with other datasets, including Basecamp Research’s proprietary BaseGraph database, to further optimise the model and improve sequence outputs.
Highlights
ZymCTRL was first trained on the BRENDA enzyme database, comprising 37m enzyme sequences.
From this, the team generated sets of carbonic anhydrases, enzymes that accelerate the conversion of carbon dioxide to bicarbonate, helping capture and store CO2, and lactate dehydrogenases, enzymes that help convert sugar into energy in our cells, with no further fine-tuning for the AI model.
After producing and purifying the proteins, several showed enzyme activity despite less than 40% of their sequences resembling proteins seen in the public database. This happened with no additional adjustments to the model.
To correct for potential biases in public databases, which have uneven sampling due a lack of biodiversity, ZymCTRL was adjusted using a wider range of lactate dehydrogenase sequences from Basecamp Research’s proprietary BaseGraph dataset.
With this fine-tuning, the team created lactate dehydrogenases with higher quality scores in silico (in computer simulations), such as better predicted local distance difference test (pLDDT) values, compared to sequences generated with no prior training.
Remarkably, active enzymes continued to show significant activity at a high temperature of 45°C as well as across a broad pH range of 4.5 to 9.5 – meaning it can work or stay stable in slightly acidic to slightly basic environments – offering significant industry advantages over naturally-occurring lactate dehydrogenases. This excellent pH tolerance allows a single enzyme to be used in many different processes with different pH levels, making the enzyme very useful and adaptable for many applications.
Two of the artificial lactate dehydrogenase enzymes were produced in larger amounts and successfully freeze-dried. They kept their activity and showed they could work in complex reactions under harsh conditions, supporting their potential for industrial use.
“Beyond the obvious excitement of being able to generate truly de novo proteins, the results are a further testament to the ability of Basecamp Research’s dataset to produce better results compared to publicly available datasets, which barely scratch the surface of the Earth’s immense biodiversity,” added Dr. Glen Gowers, Co-Founder of Basecamp Research. “Earlier we were able to show that our BaseFold model, also powered by our dataset, outperformed AlphaFold2 in predicting protein structures. Generative AI is going to have a huge impact across biotech, and we’re dedicated to collecting the data and tools needed to make its potential a reality.”