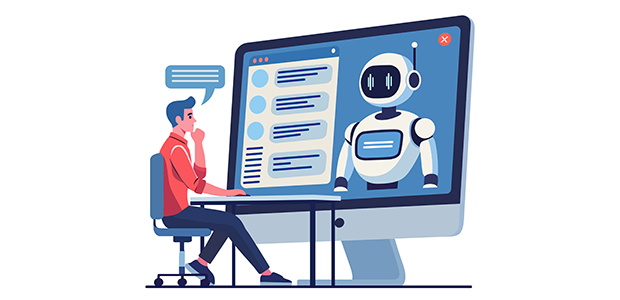
Is AI really as powerful as we think?
You have a brilliant idea for a new business, and part of the brilliance is taking advantage of where the world is now. But how long will that world remain the same? Every startup needs to consider how things might change because of curve balls such as pandemics, tariff wars, and new technology.
Whether it’s China trolling Donald Trump with AI generated videos and images of the US President and Vice President working in a shoe factory or Meta embroiled in data scraping scandals, artificial intelligence is never far from the headlines. AI triggers concern and excitement; some fear for the future of the human workforce while others see a bright future where we’re all prompt engineers. But are we getting caught up in the hype, or are we failing to see the bigger picture?
The short-term hype vs reality
According to Amara's Law, we tend to overestimate a technology's impact in the short term while underestimating its effects in the long run.
Take autonomous vehicles, for instance. Despite billions in investment and countless promises, they still struggle with the chaos of real-world roads. These systems, while impressive at specific tasks like playing games or predicting protein folding, still stumble when faced with the unpredictable complexity of the real world. This doesn’t mean, however, that researchers and practitioners have stopped chipping way. Quite the opposite. It’s just that the results are not visible. Yet.
The Hype Cycle
This pattern of inflated expectations followed by reality checks isn't unique to AI. It closely mirrors what's known as the Gartner Hype Cycle, where new technologies typically go through several phases: initial excitement, overinflated expectations, disillusionment when those expectations aren't immediately met, and finally, a steady climb toward practical productivity.
We've seen this pattern before with Cloud computing. It took seven years before serious competition emerged in the Cloud space, and only now is Cloud computing emerging from what Gartner calls the "trough of disillusionment" into practical, widespread adoption.
The journey has been fascinating to watch: AWS launched its first services in 2006, but it wasn't until 2013 that serious competitors like Microsoft Azure and Google Cloud Platform began to gain real traction.
Today, Cloud computing is delivering tangible benefits that were once just promises. These days companies like Spotify really are handling millions of simultaneous users with dynamic scaling. Netflix really does stream billions of hours of content globally. Even traditional industries are seeing results; banks are processing transactions faster, manufacturers are optimising supply chains in real-time, and retailers are delivering personalised shopping experiences at scale.
Parallel with Cloud Native
Cloud computing offers valuable insights into AI's potential path. For Cloud computing to deliver real benefits, it required more than just technology – it demanded good management, psychologically safe environments, excellent engineering skills, mature HR practices, and sophisticated financial planning. These same ingredients will be crucial for making AI productive in enterprise settings.
Let's break down what these requirements really mean, and why they're crucial for both Cloud and AI success:
Good management in Cloud computing requires knowing which real-world business problems Cloud-native can actually solve, not merely chasing tech trends. It is the same for AI. Its success hinges on leadership capable of identifying the genuine business challenges where AI can add value.
For instance, while a retailer might be tempted to implement AI chatbots because
competitors have them, good management would first identify whether customer service is actually a pain point worth solving.
Psychologically safe environments have proven essential for Cloud adoption, where teams are given the freedom to experiment and to learn from missteps. This is even more critical for AI development.
Take Netflix's approach: their culture of calculated risk-taking enabled them to successfully transition to Cloud infrastructure, and now powers their AI-driven recommendation engine development.
Excellence in engineering for Cloud means having teams that understand both technical implementation and business impact – not just how to deploy containers, but why and when they're the right solution.
For AI, this translates to engineers who can both develop models and understand their real-world applications and limitations. They need to grasp not just how to implement a machine learning algorithm, but when it's the appropriate solution for a business problem.
Mature HR practices in Cloud computing focus on finding and nurturing talent that can bridge technical expertise with business acumen. For AI development, this becomes even more crucial – organisations need people who can translate between AI capabilities and business needs, understand the ethical implications of AI deployment, and adapt to rapidly evolving technical requirements.
This might mean hiring data scientists who can explain complex models to business stakeholders, or training existing staff to work alongside AI systems.
Sophisticated financial planning for Cloud involves balancing upfront investment with long-term operational benefits, understanding the true cost of Cloud infrastructure beyond just server expenses. With AI, this becomes more complex – organisations need to account for not just computing resources, but also data acquisition and cleaning, model training costs, and the ongoing expense of keeping AI systems current and relevant.
A healthcare provider, for example, needs to consider not just the cost of AI infrastructure, but also data governance, model retraining in order to keep up to date with the very latest healthcare developments, and the potential ROI of improved patient outcomes.
Yet, unlike Cloud computing, which took decades to reach maturity, AI's development cycle is likely to be considerably shorter – perhaps less than 20 years. The way it captures the public imagination makes AI's potential applications more immediately apparent, driving massive investment.
The infrastructure question
What's really interesting in the parallels between AI and Cloud Computing is that successful AI implementation depends heavily on solid Cloud infrastructure. Without robust Cloud systems in place, organisations face significant challenges in leveraging AI effectively.
Companies with mature Cloud infrastructure enjoy substantial advantages in their AI journey. They can train AI models on their own data, creating unique competitive edges that set them apart from competitors. Their ability to scale computing resources dynamically means they can experiment freely, ramping up resources for intensive training periods and scaling back during quieter times.
Perhaps most importantly, they maintain control over their AI development roadmap, integrating AI capabilities directly into existing applications and services in ways that make sense for their specific business needs.
In contrast, organisations without strong Cloud foundations often find themselves dependent on AI-as-a-Service from cloud providers. While this might seem like an easy solution, it comes with hidden costs and limitations that can significantly impact long-term success.
As usage grows, operational expenses tend to climb steadily, often exceeding initial estimates. The inability to deeply customise AI models for specific business needs can leave companies struggling to differentiate themselves in the market, especially when competitors have access to the same off-the-shelf solutions. Moreover, being dependent on provider roadmaps and pricing strategies can limit innovation and agility – precisely the qualities that AI implementation should enhance.
The long-term perspective
While we might be overestimating AI's immediate impact, we're likely underestimating its long-term transformative potential. Away from the headlines about artificial general intelligence and robot overlords, the real revolution is happening in more subtle ways.
There are likely to be systematic changes in how work is organised and automated, with AI assistants augmenting human decision-making in complex scenarios while automated systems handle more routine tasks. This will evolve into new collaborative workflows, combining human insight with AI processing power, eventually leading to AI-first organisational structures and processes.
The job market is already beginning to shift in response to AI's influence. We're seeing growing demand for AI literacy across all roles, from marketing specialists who need to understand recommendation engines to manufacturing managers who work with predictive maintenance systems.
Traditional roles are evolving to incorporate AI collaboration skills, while entirely new positions are emerging – AI trainers, ethics officers, and AI-human interaction designers. Perhaps most importantly, there's a growing emphasis on uniquely human skills that complement AI capabilities, such as creative problem-solving, emotional intelligence, and ethical decision-making.
Business models are transforming too, as organisations discover new ways to create value with AI. We're moving from standardised offerings to highly personalised products and services, enabled by AI's ability to process and act on individual customer data at scale.
Companies are discovering new revenue streams based on AI-driven insights and predictions, while traditional industries are being transformed through AI integration. Entirely new markets are emerging, built on capabilities that simply weren't possible before.
The way we solve problems is also undergoing a fundamental shift. AI is enabling real-time optimisation of complex systems like supply chains and energy grids, making it possible to respond to changes and disruptions almost instantly.
Predictive maintenance is reducing downtime and extending equipment life, while data-driven decision making at unprecedented scales is helping organisations navigate increasingly complex business environments. Perhaps most excitingly, we're starting to see novel solutions to problems that were previously considered intractable.